Contextual Data Analysis: What is It and How Can Software Help?
DataWalk enables deeper insights through robust contextual data analysis by connecting multiple data sources.
- Data Integration. Contextual data is often integrated with the primary dataset. This can involve combining multiple data sources or enriching the existing data with additional contextual information. Integration ensures that relevant contextual factors are readily available for analysis.
- Visualization. Visualizing data along with its contextual information can help identify patterns, relationships, and trends. Visualization techniques like charts, graphs, maps, or interactive dashboards allow analysts to explore the data in a visual context, making it easier to comprehend complex relationships and draw meaningful conclusions.
- Comparative Analysis. Contextual data analysis often involves comparing different data points or groups within specific contexts. By examining similarities, differences, or trends across contextual dimensions, analysts can identify variations, anomalies, or correlations that may provide valuable insights.
- Statistical Analysis. Statistical techniques are commonly used to analyze contextual data. Statistical tests, regression analysis, correlation analysis, or clustering algorithms can be applied to determine relationships, significance, or patterns within the data while considering the relevant contextual factors.
- Machine Learning and AI. Machine learning algorithms can be trained to analyze contextual data and identify patterns, make predictions, or generate recommendations. By feeding the algorithms with contextual information alongside the primary data, models can learn to recognize and leverage the context for analysis purposes.
- Qualitative Analysis. In some cases, contextual data analysis involves qualitative methods such as content analysis, thematic analysis, or discourse analysis. These techniques focus on examining textual or qualitative data within its context to uncover underlying meanings, themes, or perspectives.
The specific techniques and methods used for contextual data analysis depend on the nature of the data, the contextual factors of interest, and the objectives of the analysis.
Common Use Cases for Contextual Data Analysis
Contextual data analysis is used in a number of different industries—including banking and finance, law enforcement, retail, insurance, national intelligence, defense, and cryptocurrency. Some of the primary use cases in these industries include:
Banking and Finance
- Transaction Monitoring. Contextual data analysis is an essential tool in monitoring financial transactions for fraudulent activities. By considering factors such as transaction amounts, frequency, velocity, geographical location, and behavioral patterns, analysts conducting this sort of analysis can identify anomalies that might indicate fraudulent behavior.
- Customer Behavior Analysis. Analyzing customer data within the context of historical behavior, account activity, spending patterns, and geographic location helps analysts to detect unusual or suspicious financial activities. Contextual analysis enables analysts to identify potential fraud, including account takeovers or identity theft.
- Anti-Money Laundering (AML). Contextual data analysis plays an increasingly crucial role in identifying potential money laundering activities. By analyzing transactional data, customer profiles, and contextual factors like transaction patterns, geographic relationships, and high-risk regions, banks can flag suspicious transactions and improve their compliance with AML regulations.
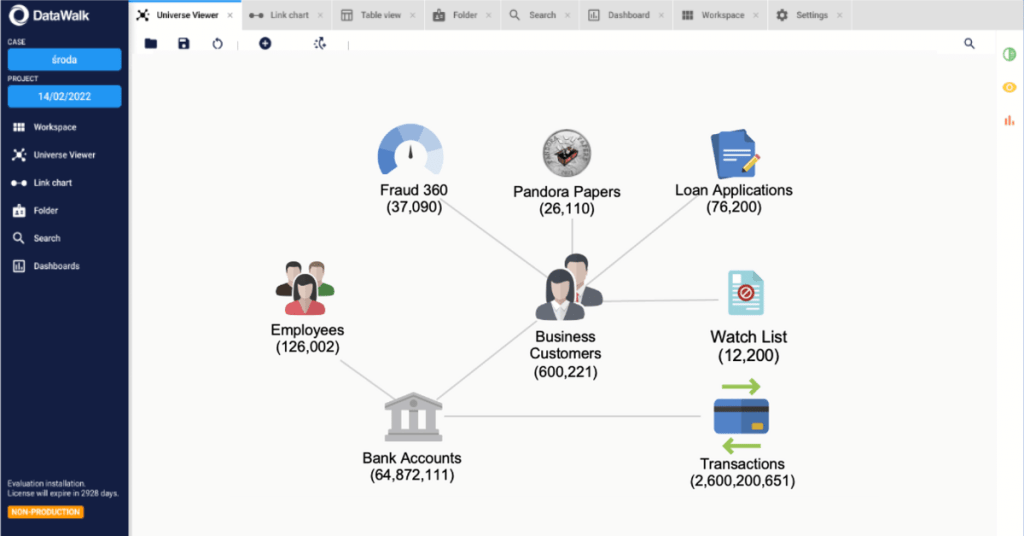
As a form of contextual data analysis, financial institutions often use knowledge graphs to link disparate data sources, revealing insights into the profiles and networks of customers, counterparties, suppliers, and their associated risks.
Law Enforcement
- Crime Pattern Analysis. Law enforcement agencies use contextual data analysis to identify crime patterns and hotspots. By analyzing crime data alongside contextual factors such as time, location, demographic information, and social factors, these agencies can allocate resources more effectively, identify trends, and better target their crime prevention strategies.
- Investigative Analysis: Contextual data analysis supports investigations by uncovering connections, relationships, and patterns among vast amounts of data. By considering contextual factors like communication networks, financial transactions, travel records, and social media interactions, law enforcement agencies can potentially generate more leads, identify suspects, and build stronger cases than without this type of analysis.
Insurance
- Claims Analysis. Contextual data analysis helps insurance companies to identify insurance claims likely to be fraudulent by examining contextual factors such as claim history, policy details, accident reports, medical records, and relationships between parties involved. Anomalies, patterns, and inconsistencies in the data can be detected, flagging potentially fraudulent claims for further investigation.
- Social Network Analysis. Analyzing social connections and relationships within insurance claims data can help analysts to reveal networks of fraudsters collaborating to stage accidents, falsify claims, or engage in organized fraud schemes.
Retail
- Point of Sale (POS) Analysis. In the retail sector, contextual data analysis assists in detecting fraud at the point of sale. By analyzing transaction data, including product details, payment methods, customer profiles, and contextual factors like store location or time of day, retailers can better identify suspicious patterns such as unusual refunds, chargebacks, or inventory shrinkage.
- Social Media Monitoring. Contextual analysis of social media platforms can provide insights into potential retail fraud. By monitoring discussions, reviews, or posts related to products, brands, or promotions, retailers can detect patterns of fake reviews, counterfeit product sales, or organized retail crime.
National Intelligence and Defense
- Threat Detection. In their efforts to detect and mitigate threats to national security, intelligence agencies analyze contextual data from various sources of information, such as social media, satellite imagery, financial transactions, and communication networks. By considering contextual factors, patterns, and anomalies, intel agencies can identify potential risks and take appropriate measures.
- Situational Awareness. Similarly, defense agencies employ contextual data analysis to maintain situational awareness. By integrating data from multiple sources, including sensors, intelligence reports, and historical data, defense analysts can understand the context of military operations, monitor developments, and make more informed decisions in real time.
Cryptocurrency
- Market Analysis. With the rise of digital currency, contextual data analysis has become essential in analyzing cryptocurrency markets. By considering factors such as trading volumes, price movements, social media sentiment, regulatory changes, and macroeconomic conditions, analysts can understand market trends, identify investment opportunities, and assess risks.
- Fraud Detection. As in other areas of finance, contextual data analysis is used in the cryptocurrency domain to help detect fraudulent activities. By analyzing transaction patterns, wallet addresses, network behavior, and contextual factors like suspicious IP addresses or known scam schemes, fraud detection systems can identify and prevent fraudulent transactions.
One example of contextual data analysis used either by banks for anti-money laundering or in law enforcement investigations, is to identify activities associated with different types of criminal behavior. In such an example, an analyst might target a series of financial transactions just under $10,000 (often indicative of money movement structured to avoid detection) that have been carried out by individuals identified as having engaged in other types of suspicious activities in the past. In this case, the transactions may be covering up proceeds from crimes such as human trafficking or assault weapons sales. Through contextual data analysis, not only does the relationship among these individuals and activities become clearer, but other significant connections might emerge, such as a common address or phone number shared by multiple people. As a result, you can more effectively identify high value targets for focus in a full scale investigation.
Software Applications That Support Contextual Data Analysis
Regardless of the industry or use case, without the right tools contextual data analysis is often complex, resource intensive, and even impractical. This has given rise to the use of advanced software tools and technologies that provide powerful capabilities for analyzing contextual data more effectively and cost-efficiently.
- Business Intelligence Tools allow users to visualize and analyze data in the context of different dimensions, helping organizations to gain insights and make data-driven decisions.
- Machine Learning and AI Platforms offer libraries and algorithms that enable the data to be analyzed in various contexts. These platforms help extract meaningful patterns and insights from large and complex datasets.
- Natural Language Processing (NLP) Libraries provide tools and algorithms for the contextual analysis of text data. They enable sentiment analysis, topic modeling, and other forms of text mining.
In addition to providing these capabilities, DataWalk, as a graph analytics application, is particularly well-suited to support contextual data analysis. Specifically designed for exploring and analyzing graph-based data (a type of data representation that captures relationships or connections between entities), DataWalk enables users to uncover valuable insights within the context of interconnected data. The software accomplishes this through several means:
- Relationship Representation. DataWalk provides a natural and intuitive way to represent relationships and dependencies between entities. Contextual data often involves understanding the connections and interactions between various factors, and graph models excel at capturing and visualizing these relationships. The graphical representation helps analysts to identify patterns, clusters, and contextual dependencies that may not be immediately apparent in other data representations.
- Contextual Exploration. DataWalk allows analysts to visually explore and navigate the graph, gaining insights into the contextual relationships between entities. By traversing the graph, analysts can gain a deeper understanding of how different entities are connected and how contextual factors influence their interactions, ultimately helping you uncover valuable insights.
- Contextual Querying. With its support for visual querying, DataWalk enables analysts to formulate queries that retrieve data based on contextual conditions and relationships. For example, you can find entities related to a specific context or uncover paths between entities that satisfy certain contextual criteria. With this querying capability you can retrieve and analyze contextual data with greater precision.
- Graph Algorithms. DataWalk provides a range of graph algorithms designed to analyze and extract insights from the data. These algorithms leverage the graph structure to perform tasks like community detection, centrality analysis, path finding, and clustering. By applying these algorithms to the contextual data, analysts can uncover patterns, identify key influencers, detect anomalies, and generate more actionable insights.
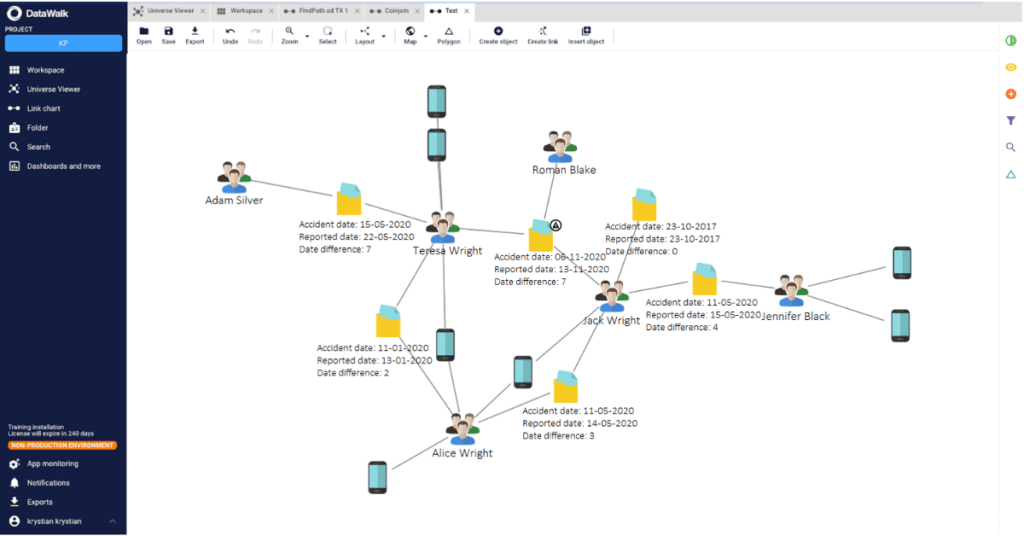
DataWalk graph algorithms enable you to perform various relationship-based analyses across all your data at the push of a button. For example, you can automatically find the shortest paths between distant entities, or automatically identify clusters that have the characteristics of organized crime groups or other networks of interest.
- Knowledge Graph Integration. A knowledge graph capability is fully integrated into the DataWalk application. Knowledge graphs are rich semantic graphs that incorporate external knowledge sources such as ontologies, taxonomies, or domain-specific vocabularies. By integrating contextual data with knowledge graphs, analysts can leverage the additional contextual information for more accurate and comprehensive analysis. This integration enhances the depth and quality of contextual data analysis.
- Contextual Reasoning. DataWalk, especially when leveraging its knowledge graph, enables reasoning and inference techniques. By leveraging the explicit relationships and contextual information stored in the graph, analysts can infer new knowledge, make contextual inferences, and uncover implicit relationships and dependencies within the data. Contextual reasoning enhances the analysis by providing deeper insights and supporting decision-making processes.
Through these powerful capabilities, graph analytics software like DataWalk automates much of the processing behind contextual data analysis. Doing so empowers users to more effectively navigate and analyze the data, ultimately leading to more comprehensive and meaningful insights.